TLDR of Argmin's Summary of Half of the Meehl Lectures
Tags: ai
, pompousness
, Date: 2024-05-22
Over at argmin.net, Ben Recht is reflecting on Meehl's lectures on the metatheory of science, which is about how science progresses. The original lectures are fascinating but also long as well as long-winded, and I found Ben's blog series a much better read (especially since the originals are video recordings). Still, at the time of writing, with 13 blog posts covering less than half of the lectures (5/12), no self-respecting 21st century scientist can risk the time investment (equivalent to publishing 0.25 papers in machine learning) or – even worse – getting slowed down by methodological considerations.
So, here is my TLDR for the busy professional: it's all Bayes and incentives. There is no silver bullet method, and while we do questionable things for all the wrong reasons, time will clean up any mess that we make anyway.
Expanding that for slightly longer attention spans:
Theories can be disproved but cannot be proved.
We can only accumulate evidence that supports a theory.
Evidence is subjective.
All theories are wrong, but some are useful.
The utility of a theory is its only grounding in reality.
At this point, the rest is somewhat predictable; my armchair is like any other. But if you can tolerate examples and spelling out implications, read on.
We want to run convincing experiments, but what is convincing to someone depends on their beliefs about the possible hypotheses, results and what they know about our methodology (never fully specified) and us (e.g. motivations, beliefs, funding).
We choose experiments to rule out large swaths of the hypothesis space weighted by our beliefs, which might bear little resemblance to others' beliefs.
If the hypothesis space is large and we don't have very strong beliefs, it may be that we don't even think in terms of hypotheses. Instead, we may think in terms of probability of results (as if we marginalized out the hypotheses). "Hey, these results hold to 37 decimal places! What do you think the chances of that are if our model was wrong?"
The entire process that produced a result is considered in belief updates. This includes the researcher, the machinery, the funding agency, the organization, etc.
With so many factors, there is always room for different interpretations of results. Eventually, theories die when they are no longer useful (for any purpose).
Classical formal logic has limited use in this setting. It seems to be all Bayes with a bit of decision/game theory thrown in.
In these Bayesian belief updates, perceived incentives play a prominent role: many results are downweighted because we know the twisted academic and applied research incentive structure.
I believe improving the incentives is the most important contribution one can make in today's world. Now, go and read Ben's posts.
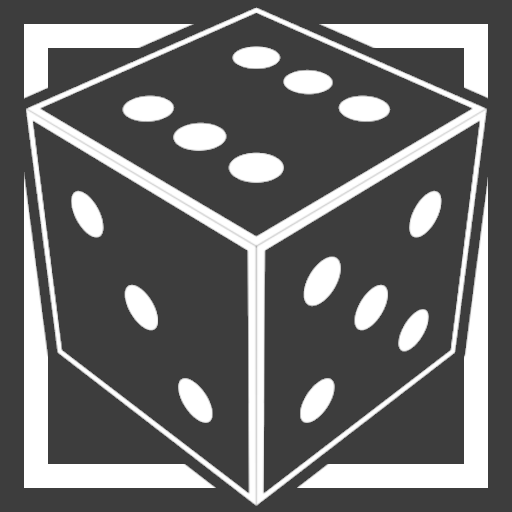